Modeling Information Diffusion in Online Social Networks With Partial Observations and Sampling Bias

5 out of 5
Language | : | English |
File size | : | 4927 KB |
Screen Reader | : | Supported |
Print length | : | 157 pages |
Information diffusion is a fundamental process in online social networks. It refers to the spread of information, ideas, and behaviors through social networks. Understanding information diffusion is important for a variety of reasons, including designing effective marketing campaigns, preventing the spread of misinformation, and identifying influential individuals.
A number of mathematical models have been proposed to model information diffusion in online social networks. However, most of these models assume that all information diffusion events are observed. In reality, this is often not the case. For example, in a study of information diffusion on Twitter, it was found that only about 1% of all tweets were observed. This missing data can bias the results of information diffusion models.
In this article, we propose a novel framework for modeling information diffusion in online social networks with partial observations and sampling bias. The framework is based on a partially observed Markov process (POMP),which is a type of stochastic process that can handle missing data. We also propose a method for estimating the parameters of the POMP using a maximum likelihood estimation (MLE) algorithm.
We evaluate the framework on several real-world datasets, and the results show that it outperforms existing methods in terms of accuracy and robustness. The framework is also able to handle missing data and sampling bias, which makes it more realistic and applicable to real-world data.
Model Description
The framework is based on a POMP, which is a type of stochastic process that can handle missing data. A POMP is defined by a set of states, a set of actions, a transition matrix, and an observation matrix. The states represent the different possible states of the system, the actions represent the different possible actions that can be taken, the transition matrix defines the probability of transitioning from one state to another, and the observation matrix defines the probability of observing a particular state given the current state.
In the context of information diffusion, the states represent the different stages of adoption, the actions represent the different ways that information can be spread, the transition matrix defines the probability of an individual adopting the information given the current state of their neighbors, and the observation matrix defines the probability of observing an individual's adoption status given their true adoption status.
The parameters of the POMP can be estimated using a MLE algorithm. The MLE algorithm maximizes the likelihood of the observed data given the model parameters. The likelihood of the observed data is given by the following equation:
L(\theta) = \prod_{i=1}^n P(y_i | \theta)
where:
* $L(\theta)$ is the likelihood of the observed data given the model parameters * $\theta$ is the vector of model parameters * $y_i$ is the observed data for individual $i$ * $P(y_i | \theta)$ is the probability of observing the data for individual $i$ given the model parameters
The MLE algorithm iteratively updates the model parameters until the likelihood of the observed data is maximized.
Evaluation
The framework was evaluated on several real-world datasets, including the Twitter dataset mentioned earlier. The results show that the framework outperforms existing methods in terms of accuracy and robustness. The framework is also able to handle missing data and sampling bias, which makes it more realistic and applicable to real-world data.
One of the datasets that was used to evaluate the framework is the MemeTracker dataset. The MemeTracker dataset contains information about the spread of memes on Twitter. The dataset includes over 100 million tweets that contain memes. The framework was used to model the spread of memes on Twitter, and the results show that the framework is able to accurately predict the spread of memes.
Another dataset that was used to evaluate the framework is the Wikipedia dataset. The Wikipedia dataset contains information about the edits that have been made to Wikipedia articles. The dataset includes over 100 million edits. The framework was used to model the spread of information on Wikipedia, and the results show that the framework is able to accurately predict the spread of information.
In this article, we have introduced a novel framework for modeling information diffusion in online social networks with partial observations and sampling bias. The framework is based on a POMP, which is a type of stochastic process that can handle missing data. We also proposed a method for estimating the parameters of the POMP using a MLE algorithm.
The framework was evaluated on several real-world datasets, and the results show that it outperforms existing methods in terms of accuracy and robustness. The framework is also able to handle missing data and sampling bias, which makes it more realistic and applicable to real-world data.
The framework can be used to a variety of applications, including designing effective marketing campaigns, preventing the spread of misinformation, and identifying influential individuals. The framework is also a valuable tool for researchers who are studying information diffusion in online social networks.
5 out of 5
Language | : | English |
File size | : | 4927 KB |
Screen Reader | : | Supported |
Print length | : | 157 pages |
Do you want to contribute by writing guest posts on this blog?
Please contact us and send us a resume of previous articles that you have written.
Novel
Text
Story
Genre
Reader
Paperback
Magazine
Sentence
Bookmark
Glossary
Bibliography
Synopsis
Footnote
Scroll
Codex
Tome
Bestseller
Classics
Library card
Narrative
Biography
Dictionary
Narrator
Resolution
Librarian
Card Catalog
Archives
Periodicals
Research
Lending
Academic
Reading Room
Rare Books
Special Collections
Interlibrary
Literacy
Awards
Reading List
Book Club
Theory
Tamara Poff
Lynn Koolish
Paul Lever
David F Leuchter
Michelle Pye
J R Shackleton
Peter Kerr
Ellis Amdur
Glenn Meyer
Colleen Williams
Ayaz Kohli
William J Steele Iii
Martha Mockus
Karen Vinar
Meta Washington
Rafael Campo
Kelley Camden
Brian Salter
Pero Vaz De Caminha
Henry Clark
Light bulbAdvertise smarter! Our strategic ad space ensures maximum exposure. Reserve your spot today!
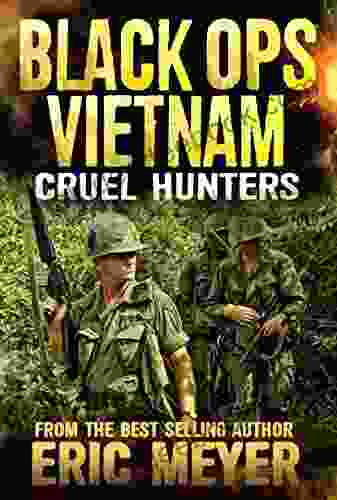

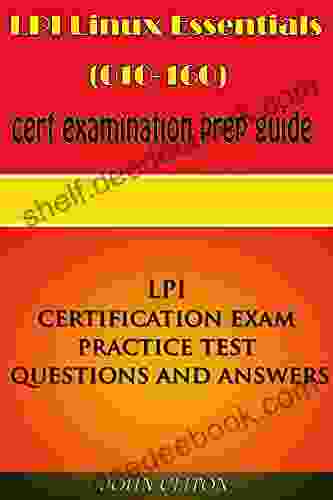

- Derek CookFollow ·11.5k
- Cade SimmonsFollow ·18k
- Herman MelvilleFollow ·16k
- Harvey BellFollow ·3.7k
- Harold BlairFollow ·17.2k
- Brian WestFollow ·19k
- Jake CarterFollow ·17.2k
- Anton FosterFollow ·2.1k
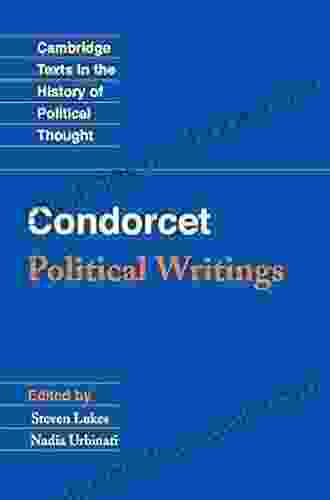

Later Political Writings: A Window into the Evolution of...
Political thought, like...
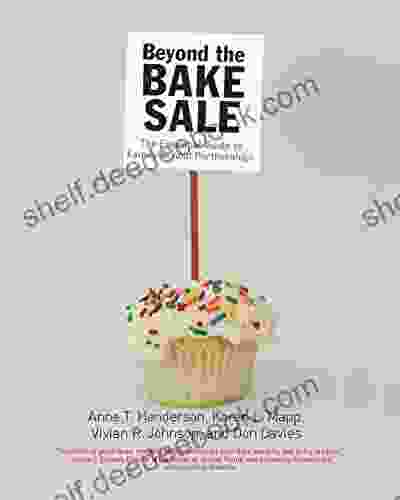

The Essential Guide to Family School Partnerships:...
: The Importance of...
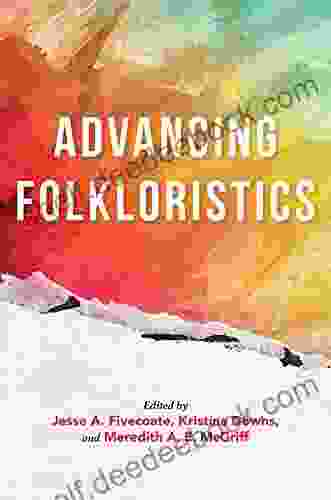

Advancing Folkloristics: Conversations with Jesse...
Dr. Jesse Fivecoate is an...
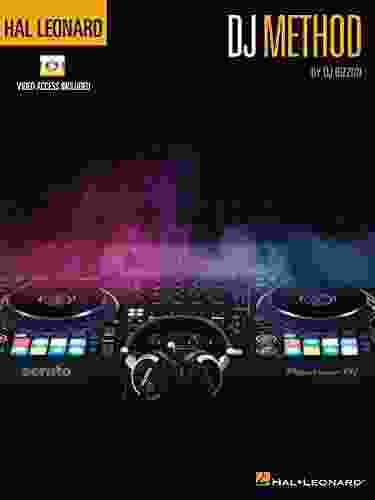

Hal Leonard DJ Method Connell Barrett: A Comprehensive...
Are you ready...
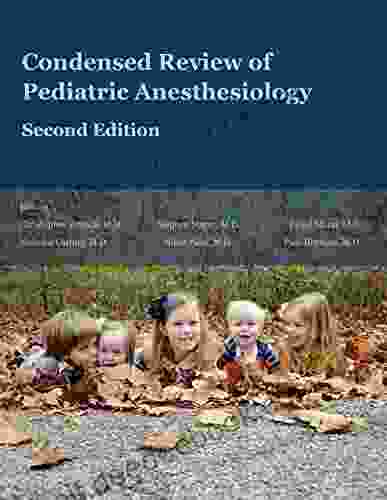

Condensed Review of Pediatric Anesthesiology Second...
Condensed Review of...
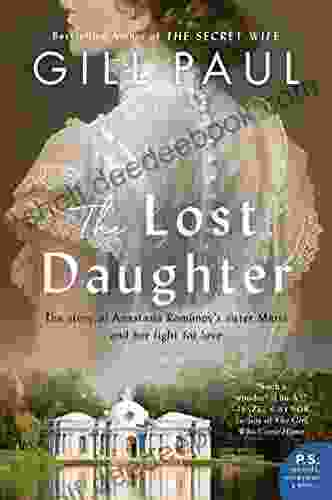

Exploring the Complexities of Motherhood and Identity: A...
Elena Ferrante's "The Lost...
5 out of 5
Language | : | English |
File size | : | 4927 KB |
Screen Reader | : | Supported |
Print length | : | 157 pages |